Recursive AI for Solar Energy Management
Helps the renewable energy sector maximize returns and reduce investment risks by optimizing site selection and improving panel performance with highly accurate AI and physics-based solar energy predictions.
Maximize Returns, Reduce Investment Risks
Renewable energy is essential for reducing carbon emissions and combating climate change. However, high investment costs and uncertainty in power output create barriers for solar energy projects.
Recursive AI for Solar Energy Management leverages satellite and topography data with machine learning and physics-based modeling to provide high-precision solar radiation and power output predictions, enabling more confident investments and efficient planning. With 96% yearly and 94% monthly accuracy in radiation predictions, it helps energy providers optimize site selection, improve operations, and accelerate the adoption of renewable energy.
Highlights
- Solar Radiation Predictions: High-resolution, accurate forecasts that reduce investment risks and support optimal site selection, even in complex terrains. The model can achieve predictions at the square-meter level when provided with detailed topographical maps.
- Power Output Forecasting: Advanced modeling integrates radiation, wind speed, and topographical data to improve energy production efficiency by accounting for real-world conditions.
- Historical Data Insights: Leverages 20 years of data for variability analysis, enabling reliable long-term planning and reducing uncertainties in energy output.
- Rapid Project Evaluation: Accelerates project assessments without sacrificing accuracy, allowing businesses to evaluate more sites and make faster, informed decisions.
Implementation
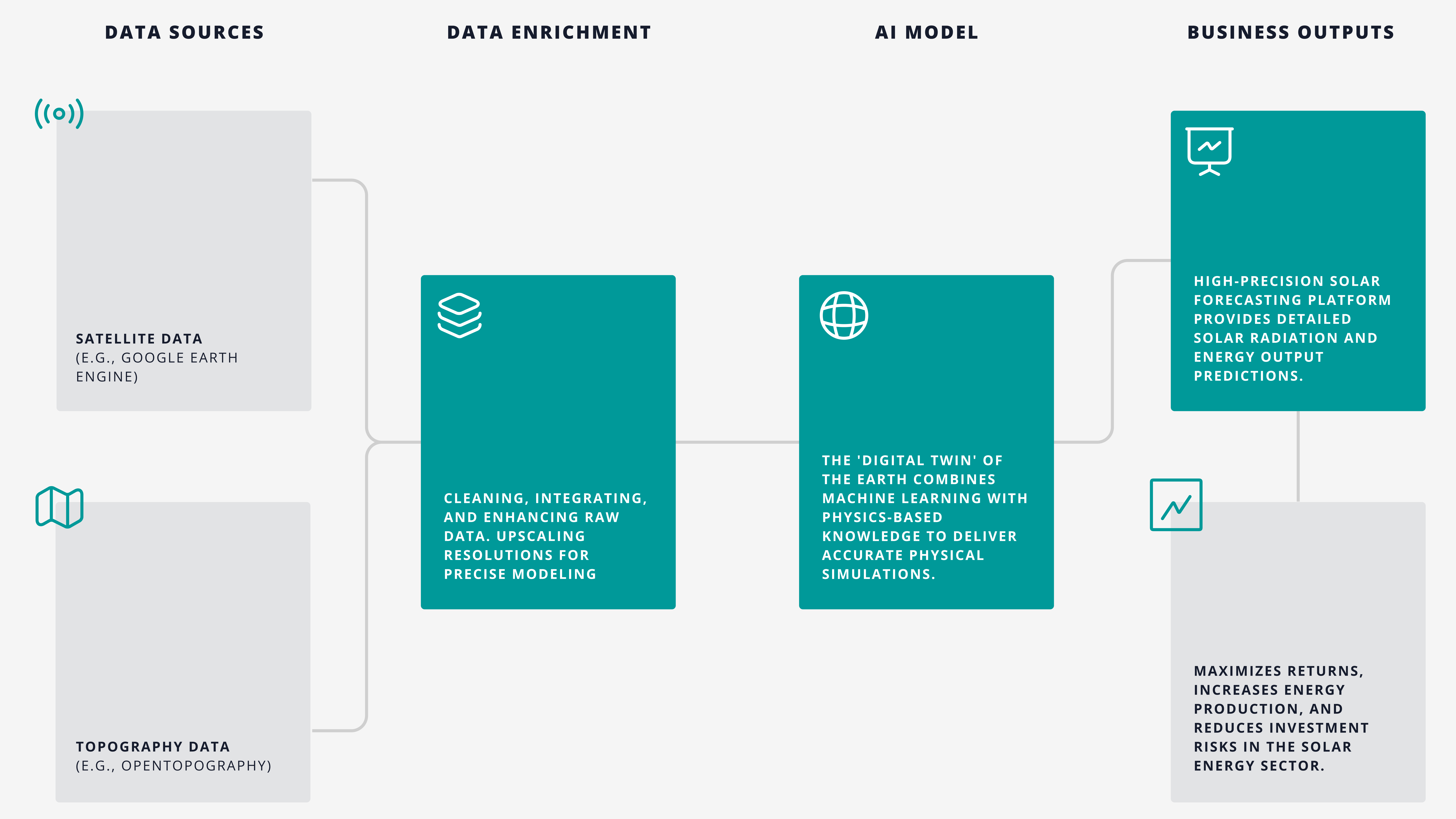
Typical implementation flow
Results within months
- 012 hours
Executive Briefing
- 022-4 weeks
Planning & Research
- 031-2 months
AI / Machine Learning Customization
- 04
Software Customization
Launch