How AI can help achieve the Sustainable Development Goals
AISDGs2022-03-11
The sustainable development goals were set in 2015 by the United Nations to guide our society towards a fairer, healthier and cleaner future.There are 17 generic goals that cover a range of areas from healthcare to clean energy to industry productivity. Progress towards each goal is quantified by a set of measurable targets. However, the UN has provided no guidance as how to achieve those targets.Governments are setting national roadmaps right now for societal scale projects that can help drive some of the targets forward, but this will not be enough. Some of the burden will fall on companies to innovate and adopt advanced technology in order to make their practices more sustainable.AI has emerged as one of the most powerful tools of the early 21st century. From increased productivity, to super-human performance in various tasks, AI can deliver solutions to problems that were before considered unsolvable. It is then fair to ask, What about sustainability?A recent study has shown that AI will be a critical ingredient to achieve the majority of targets in 16 out of 17 goals. Companies that wish to meaningfully contribute to achieving these goals will have to implement AI solutions in their workflow.In this post we will show you specifically how AI can be used to drive measurable progress towards meeting the targets set by the UN.
Higher industrial and agricultural productivity
Higher industrial productivity allows us to maintain modern standards of living with less impact on the environment. The more efficient agro-industrial production is, the less land area and natural resources we use and fewer pollution we generate.
In the agriculture business, AI is being used to monitor lifestock at a large scale, improving productivity per animal; as well as automatically identify and remove pests, which reduces the need for pesticides.
Automated food sorting devices can be used to order food items according to consumer preferences, optimizing product quality automatically. These technologies rely on automatic instance segmentation, a technology which has seen huge progress the last few years.
In industrial production, instance segmentation and classification is also an important tool used in automated quality control inspection as well as in robotics. For example a robotic tool may be able to select specific parts from a bin or measure how many items are in a specific area using vision alone.AI can also be used for assembly line integration and optimization. Legacy systems consist of individual machines optimized for a specific task, with gaps in the process filled by humans. Using techniques such as global optimization and endowing each tool with sensors, the assembly line can be completely automated and integrated.
Forecasting techniques can be applied to historical data for predictive maintenance techniques. For example, we can predict when a tool is likely to fail and preemptively deploy a maintenance team. Resources can also be intelligently redirected by an AI controller in the case of a fault to prevent a system shutdown.
Automated control systems powered by AI can also be used to optimize energy efficiency, as well as reduce pesticide usage by identifying which areas need to be sprayed or not. Similarly, food manufacturers can optimize their production processes to use less raw material and reduce calorie counts in their final products.
Logistics is another area ripe for disruption. A machine learning based algorithm can predict public transit delays with up to 30% higher accuracy than existing algorithms, while a graph neural network based system achieved up to 50% more accurate travel time predictions on Google Maps.
These algorithms can be readily applied to global supply chains and logistics networks, potentially reducing their carbon footprint as well as speeding up transit times and reducing waste of perishable goods due to delays.
Reinforcement learning is a promising technology to automate certain types of tasks that are hard to define specifically using rules or quantitive functions. This has the potential to revolutionize robotics in the manufacturing industry by freeing up humans from repetitive, but infrequent, tasks.
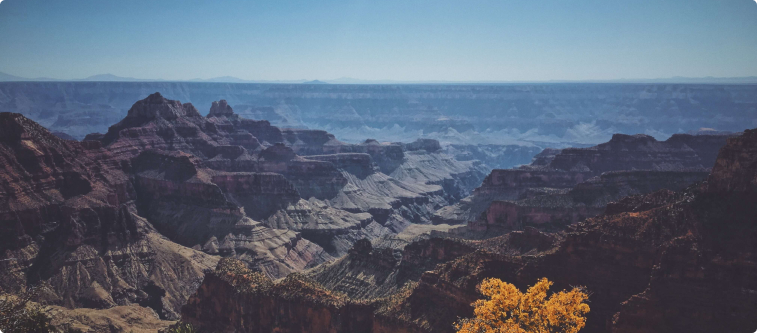
Accelerated innovation
Many of the challenges outlined in the Sustainable Development Goals will require technological innovation to achieve. Climate goals, advance healthcare, and affordable food while conserving our planet's land and oceans can be achieved through scientific and engineering breakthroughs enabled by AI.In the field of healthcare, big data and AI can unlock the next generation of drugs via data-mining based drug discovery. Techniques such as graph neural networks and attention have enabled breakthroughs in protein folding, which will speed up drug research labs all over the world.In industrial applications too, physical simulations using AI can be used to deepen understanding of the physical properties of materials, as well as accelerate the discovery of completely new ones.Scientific simulation, crucial to the development of the next-generation of transportation vehicles as well as optimization of multiple industrial processes, can be accelerated by multiple orders of magnitude.
Prevention and mitigation
As natural disasters stemming from climate change occur more frequently and with more force, disaster prevention will become an increasingly critical area for policy makers.
Based on historical data, recent research has shown high accuracy in determining areas of high risk for floods and fires, as well as earthquakes. Another model was developed to predict and mitigate hazardous air pollution levels.
After a disaster has occurred, AI based image processing algorithms can analyze satellite and drone imagery of the affected areas and determine what is the extent of the damage. Such analysis can make relief logistics much faster and more efficient.
NLP based algorithms can monitor social media feeds in real time to get more precise information on the status of certain affected areas. They can also be used to deploy chatbots to make requesting help by those affected faster.
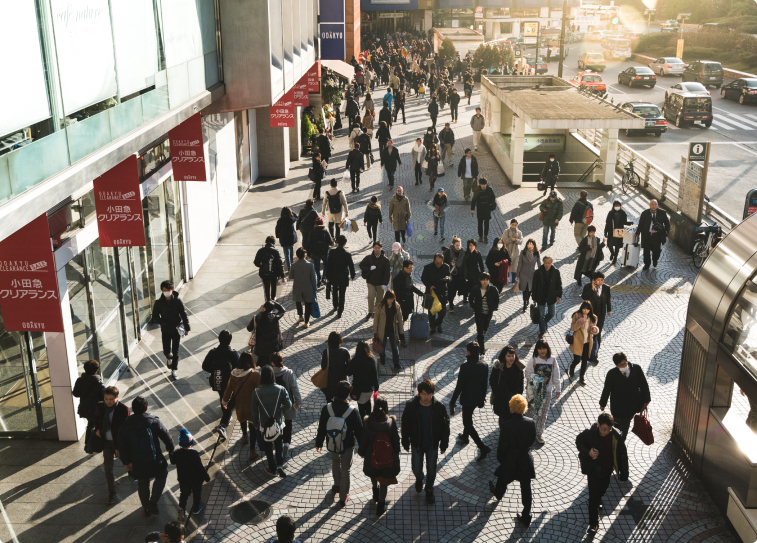
Better work and wealth distribution
While AI algorithms can achieve super-human performance on certain tasks, we know that they cannot completely replace humans. We hope that AI will serve as an enabler, freeing humans from repetitive tasks and enabling us to use our creativity to find solutions to problems we don't yet know how to solve.
To achieve this, it is important that the entirety of our workforce, no matter the background, is able to access quality education for low cost.
Automated curriculum discovery and tailored content creation tools powered by AI can deliver the next-generation in education technologies. This is particularly important for developing economies where there is the potential to democratize access to education at the level of top-tier universities.
Knowledge graph technologies combined with the latest advances in NLP can be used to create a personalized skills breakdown for every person in the workforce. This enables an individual to develop an education plan tailored to their own career goals, as well as match them with open positions that could most benefit from their existing skills.
Ethics and bias
While AI technologies have the potential to revolutionize our society and solve some of the world's biggest problems, they also pose important ethical concerns if deployed without serious consideration.
Recently AI algorithms were shown to perpetuate societal bias with respect to minorities. Among other examples, language models can learn biased associations between words describing particular ethnic or religious groups. Generative models learn to generate some face types in preference of others.
In light of these examples, it is clear that we cannot rush the deployment of AI technology as soon as we have a working prototype. A more thoughtful approach is required.
In "AI for social good" the authors outline the key principles for ethical AI development:
- Expectations of what is possible with AI need to be well-grounded.
- There is value in simple solutions.
- Applications of AI need to be inclusive and accessible, and reviewed at every stage for ethics and human rights compliance.
- Goals and use cases should be clear and well-defined.
- Deep, long-term partnerships are required to solve large problems successfully.
- Planning needs to align incentives, and factor in the limitations of both communities.
- Establishing and maintaining trust is key to overcoming organisational barriers.
- Options for reducing the development cost of AI solutions should be explored.
- Improving data readiness is key.
- Data must be processed securely, with utmost respect for human rights and privacy.
We believe these guidelines form only the minimum set of requirements, and as we learn more surely we will only add to this list. Broadening the diversity of AI developers and including the broader community in AI development is critical to this effort.
Author
Co-founder and CEO
Tiago Ramalho
Tiago holds a Master's degree in Theoretical/Mathematical Physics and a PhD in Biophysics from Ludwig-Maximilians University Munich. After graduation, he joined Google DeepMind as a research engineer. There he worked on a number cutting-edge research projects which led to publications in international machine learning conferences and scientific journals such as Nature. He then joined Cogent Labs, a multinational Tokyo based AI start-up, as a lead research scientist. In August 2020 co-founded Recursive Inc, and is currently CEO.