DeepSeek: A Game Changer in AI Efficiency and Cost?
AIGenerative AI2025-02-05
Since ChatGPT's release in November 2022, the race among tech giants to develop bigger and better large language models (LLMs) has been so intense that a new model launch rarely shakes the industry. However, DeepSeek’s latest release has done just that. The Chinese AI startup has introduced R1, an advanced reasoning model that delivers high-level performance at a fraction of the usual cost.
DeepSeek initially reported that its model cost just $6 million to train, significantly lower than what AI giants like OpenAI and Google typically spend. It was later revealed, though, that this figure only accounted for the final training run, while the full training process requires multiple iterations.
Despite this, the claim alone triggered a sharp selloff in AI-related stocks, with Nvidia losing nearly $600 billion in market value in a single day—the largest one-day loss for any public company. However, the market quickly rebounded, with Nvidia recovering nearly half of its losses within 24 hours.
While we will leave financial analysis and predictions aside, this blog post will explore what makes DeepSeek-R1 stand out and how its open-source nature is set to accelerate AI advancements in performance, hardware requirements, and cost structures.
What Makes DeepSeek-R1 Stand Out?
DeepSeek-R1 has gained attention for two key reasons: its technical advancements and open-source accessibility. Unlike OpenAI and other companies that keep their model training processes proprietary, DeepSeek has made its code and training data publicly available, enabling researchers and smaller enterprises to build on its innovations without requiring billion-dollar investments.
So, what technology makes DeepSeek so powerful that it can rival OpenAI’s most advanced model?
Test-Time Compute
Traditional LLMs process queries with static inference, meaning they use the same amount of computational effort for every task, whether it's answering a simple factual question like "What is the capital of Japan?" or tackling a complex, multi-step reasoning problem like "Explain the economic implications of climate change." This rigid approach works well for straightforward tasks but struggles with deeper analytical reasoning.
Test-Time Compute (TTC) changes this by enabling dynamic resource allocation based on task complexity. If a query is simple, the model retrieves an answer quickly with minimal processing. However, for complex problems, it can "pause and think"—allocating additional resources to run extra calculations, iterate through reasoning steps, and refine its output before giving the final response.
This mechanism mimics how humans think. When answering basic questions, we rely on quick recall, but for complex problems, we take time to analyze, break down information, and piece together a well-thought-out response.
A key component of this process is Reward Modeling, which helps the model rank and evaluate multiple outputs before selecting the final answer. Instead of generating a single response, the model produces several candidates, each representing a possible solution. A reward model then scores these outputs based on accuracy, coherence, and logical consistency. The highest-ranked response is selected, ensuring the final output is more relevant, well-reasoned, and aligned with the intended objective.
While OpenAI o1 and Gemini 2.0 Flash Thinking have incorporated Test-Time Compute, DeepSeek-R1 is the first open-source model to leverage this approach, making advanced reasoning capabilities more accessible to researchers and developers worldwide.
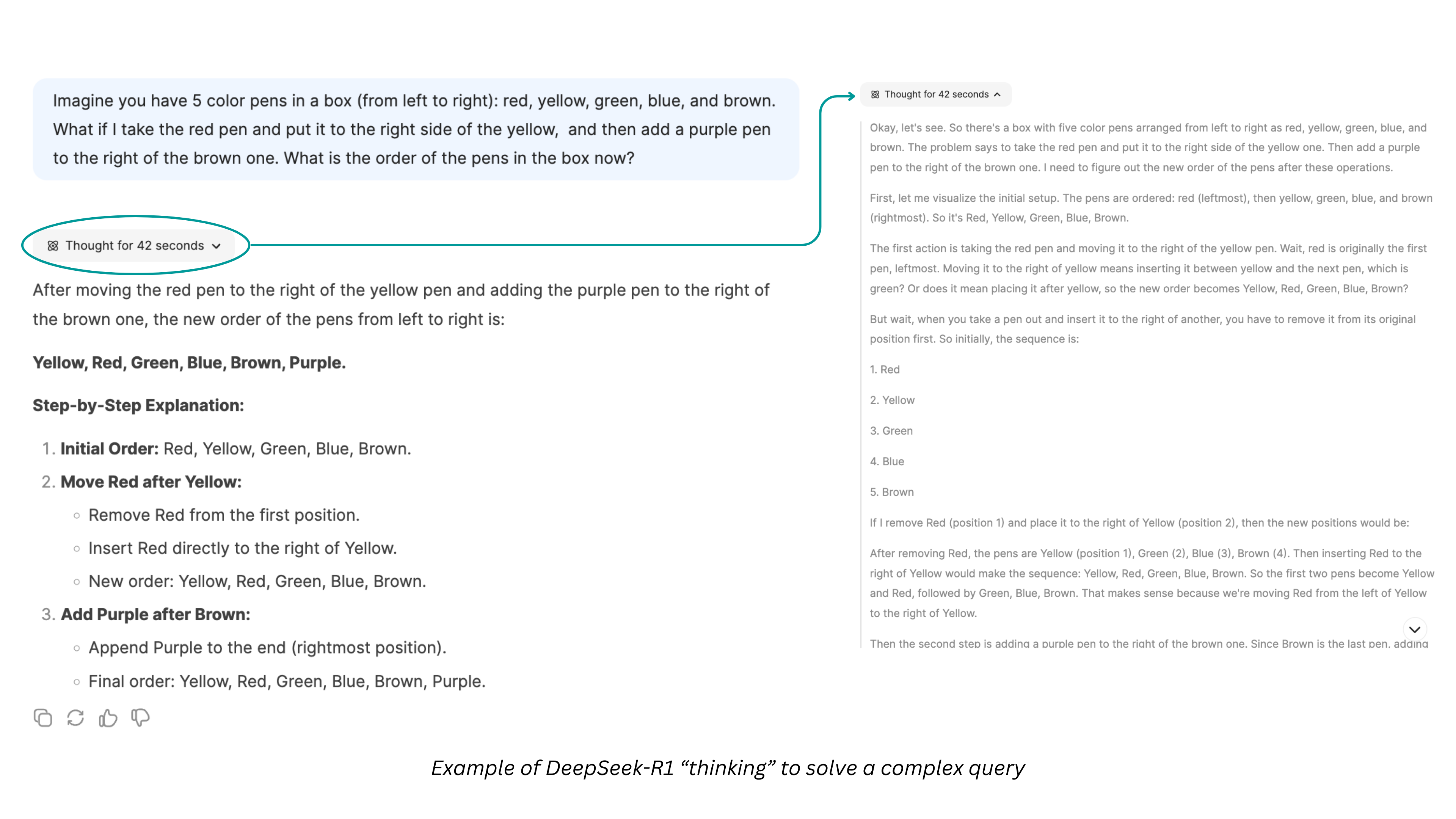
Mixture of Experts
Another key technology that helps DeepSeek models reduce computational costs and improve efficiency is the Mixture of Experts (MoE) architecture. While not new, MoE was already incorporated into DeepSeek’s previous V3 model and has been adopted in other open-source models. In 2023, the French AI company Mistral released its MoE-based open-source models, Mixtral 8x7B and Mixtral 8x22B, further demonstrating the growing adoption of this approach.
So how does it work? Traditional LLMs with hundreds of billions of parameters generate tokens at a very high computational cost because they activate the entire model for every query—even when only a small portion of the network is actually needed. This leads to unnecessary resource consumption, making large-scale AI models expensive to run and difficult to deploy on smaller devices.
MoE solves this inefficiency by dividing the model into specialized "expert" subnetworks and activating only the most relevant experts for each input token. A gating network dynamically selects which experts should handle the query, ensuring efficient resource allocation. Instead of activating all 671 billion parameters in DeepSeek for every query, MoE selects only a small subset—around 37 billion parameters—based on the task at hand.

This selective activation dramatically reduces computational costs and GPU requirements, making the model cheaper to run and enabling it to operate on smaller devices. Imagine running a model comparable to ChatGPT-4o on your personal computer at home, with full access to its code and training data—all while paying 4.5 times less per token than ChatGPT-4o.
This accessibility gives DeepSeek’s models a strong competitive advantage, allowing startups and independent researchers to fine-tune them and develop powerful AI solutions without requiring massive infrastructure investments.
A Real Breakthrough or a Predictable Evolution?
While these techniques effectively work together to achieve high performance at a fraction of the cost, they are not entirely new. The greatest advantage of the open-source ecosystem is that every new model can be studied, refined, and fine-tuned by engineers worldwide, leading to continuous improvements. This cycle of innovation has driven significant advancements in open LLMs over the past few years, making them smarter, faster, and capable of running on smaller devices.
Check out our recent articles on the best-performing open-source LLMs and fine-tuning small LLMs to achieve high performance with fewer resources.
Give it another month—and companies like Meta or Google will likely release a similar or even better open-source model. What made DeepSeek such a disruptive force wasn’t just its technical achievements but the fact that it was developed far outside of Silicon Valley—in China, where AI chip exports have been restricted by U.S. regulations since 2022.
Overall, DeepSeek’s release highlights 2 important trends in AI development:
- AI is no longer just about building bigger models but about smarter training techniques that improve efficiency. Companies like Meta, Google, and Alibaba have already released open-source models as small as three billion parameters, which can be fine-tuned for improved performance. We are inevitably moving toward a future where high-performance LLMs can run even on smartphones.
- The shift toward open-source technology enables faster, cost-effective, and transparent AI development, fostering innovation beyond the monopoly of a few major players. This aligns with Recursive’s mission of applying AI for sustainability by making knowledge more accessible worldwide. The open-source ecosystem allows us to fine-tune LLMs to account for different languages and cultural uniqueness, ensuring AI serves a broader and more inclusive audience.
At Recursive, we support the growth of open-source AI and continuously leverage the latest research and technological advancements to develop AI solutions for sustainable growth across industries. If you're interested in exploring how these solutions can enhance your business, get in touch with our team.
Author
Marketing and PR Associate
Alina Paniuta
Originally from Ukraine, Alina earned her Master’s degree in International Communications from National Chengchi University (Taiwan). After four years in Taiwan working as a Marketing Manager, she relocated to Japan in 2024 to join the Recursive team and experience life in her dream country.