Speeding up drug development using AI
AI2022-03-15
The field of modern medicine is ripe for disruption. Biological systems are complex, high-dimensional and hard to describe using traditional scientific methods. Up to now, progress has been slow since each step forward requires deep empirical investigation powered by human trial and error. The emergence of powerful computers is starting to revolutionize this field by enabling large-scale simulations of biological systems, as well as accelerating drug targeting and discovery based on historical data using AI algorithms.
Drug development is an interesting case study that illustrates how AI is not a tool that can replace an innovation pipeline end-to-end. Rather, we need to consider the pipeline’s purpose and all its different steps in order to identify certain parts that can be replaced by AI systems. While incredibly powerful at discovering hidden patterns in data, AI algorithms are best applied to specific problem domains where a quantitative optimization objective can be defined.
Drug development pipeline
The drug development pipeline is an excellent example where we see AI’s pattern matching and high dimensional optimization complement the human ability to think creatively and direct discovery based on real world goals. If we break this pipeline down into its core components we find that there are a number of different steps that come into play for drug discovery.
Target identification
To develop an effective treatment for a disease, we need to find molecules that are relevant to that disease’s mechanism, and understand their structure so that we can design a drug that can attach to them and modify their behavior. For example in the case of a bacterial or viral infection, we would need to characterise molecules specific to those pathogens that the drug can attach to and how that drug might prevent their replication. This can be done in silico (computer simulation) but it most often needs to be confirmed in vitro, that is, a test system for the drug in a real live biological system.
So how can AI help speed up this stage in the development pipeline? The very first step is target validation, which means that we need to identify targets in a biological system that could be disrupted by certain molecules; for now this is a human task that relies on the mechanistic understanding of the biology of the system, but our knowledge of biology is still very rudimentary. Unlike in other physical systems, it is difficult to isolate specific components of a regulatory pathway involved in a single process, because natural selection tends to create systems that are highly entangled and with a high level of reuse, creating very complex systems that are hard to characterize.
To improve our understanding of this process, AI systems can be used to reverse engineer the regulatory networks in cells from experimental data. Using high-throughput assaying systems to measure multiple perturbed cell cultures, we can collect enough data variation and quantity to create a model of the regulatory network that is most plausible to have generated that data. Using that model, we can understand which perturbations in the network would result in a change in the disease progression.
Once we know where in the molecular pathways we want to perturb with a drug, it is useful to know what is the molecular structure of the proteins involved. In many cases, we know the genetic code for the proteins, but we don’t know what is its final molecular structure inside a cell. It can be quite difficult to obtain this structure as it requires a complicated experiment where the molecules must be crystallized and then photographed with x-rays. In some cases this process damages the proteins and it can take years until researchers develop the right protocol to capture this structure correctly for a single protein.AI techniques such as AlphaFold 2 can greatly accelerate this process by predicting the protein structure from the genetic code. The model was trained on known genetic sequences and their corresponding protein structures, and can generalize to novel, unseen sequences. Even if not 100% accurate, these predictions can greatly accelerate the discovery process as experimental teams no longer need to wait years for each new protein to be characterized via crystallography experiments.
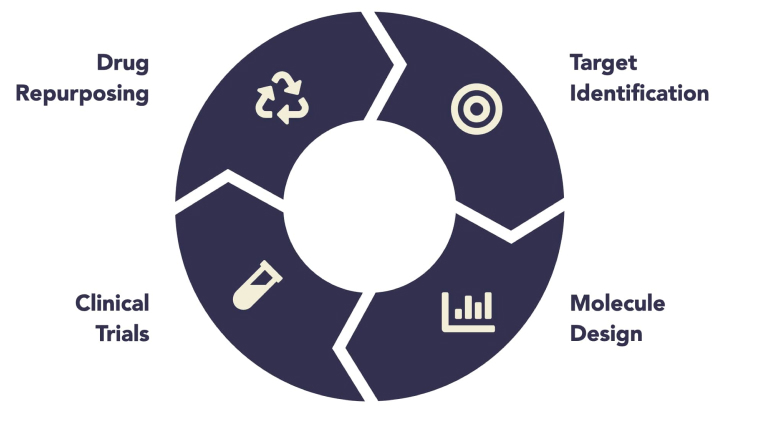
Molecular design and optimization
We then need to find compounds that could potentially interact with the molecular pathways we’ve identified in such a way as to prevent or modify the course of a disease. Their efficacy must be tested empirically by synthesizing different molecules and testing them in silico or in vitro. After identifying promising drug candidates, it will be necessary to optimize their pharmacological properties to minimize side-effects and maximise their half-life in the body.
The identification of candidate compounds can be sped up by a number of mechanisms:
- Molecule-based generative models can propose novel molecule candidates without human guidance. These AI systems learn to predict the structure of existing drug molecules and then generalize to predicting novel molecule configurations that have similar properties to known compounds. Such systems have been used to generate novel candidates for antibiotics.
- More directly, we can design models that predict perturbations at the single cell level without explicitly modelling the regulatory pathways. That means the machine learning model learns to model the cellular response to a certain perturbation by learning from a dataset of cell responses to similar molecules. With an accurate enough system, we can perform a quick computational screen of which compounds are likely to have an effect and which ones are not.
- Some groups have also made progress in modelling drug and target interactions with less mechanistic understanding, relying instead on statistics. By leveraging data on existing drugs and their targets, it is possible to train models that predict the affinity of a given drug-target pair. Such models can be used to identify previously unexplored targets for known molecules, or generate novel molecule designs given a specific target.
Beyond proposing candidate molecules for novel drugs, AI systems can also help in optimizing other pieces of the molecular design pipeline. For example, classification models can be used to predict pharmacological properties such as the half-life in the body or side-effects and drug interactions directly from the molecular structure.
Reinforcement Learning can be a useful tool to create agents to automate the experiment design for in vitro testing, by automatically integrating experimental data and proposing novel configurations to test based on the historical experiment results.
Clinical trials
If a drug candidate shows success in in vitro and animal models, it can move on to the clinical trial stage which is broken down into three phases: phase I which checks for safety; phase II which tests for preliminary efficacy; and phase III where effectiveness in a large population must be demonstrated for approval. After drug approval, ongoing surveillance tests for any rare effects and risk/benefit trade-offs.AI systems can help accelerate clinical trials in a variety of ways. Improved data collection and record keeping systems using NLP and computer vision systems can prevent human error and accelerate data input. Adverse events can be immediately flagged and reported to prevent additional harm in the case of unexpected side-effects.
Participant recruitment can be improved by ensuring that the population is well sampled, accounting for ethnic and other types of diversity. Genetic targeting and disease phenotyping using high-throughput sequencing and other technologies can be used to narrow down the range of participants to ones where the drug is more likely to have an effect, helping to show effectiveness more quickly in a smaller sample size. This kind of targeting might also allow us to confirm effectiveness in drugs that might otherwise be deemed ineffective by narrowing their use down to a more specific cohort, which is the promise of personalized medicine.
Drug repurposing
Given the vast number of effective compounds already on the market, a relatively cheap and efficient way to find new treatments is via drug repurposing. By using drug-target affinity models as we described above, we can identify potential new targets for existing drugs that are already known to be safe and not have major side-effects. This can shorten the discovery cycle as well as vastly accelerate the time needed to perform clinical trials for a specific drug.
Drug repurposing has recently shown to be effective in helping advance drug discovery for the recent COVID-19 pandemic. A traditional drug research pipeline could not deliver results in time to respond quickly and effectively to an emerging epidemic, and drug repurposing using machine learning techniques has provided a lifeline to identifying promising therapies in a span of months, compared to years. While current efforts have had mixed results, a recent study has shown that lessons learned from this epidemic will greatly accelerate future drug repurposing initiatives.
Overall, we believe that AI accelerated drug development has the potential to transform the field of healthcare over the coming decades with a faster experimentation and design cycle for more drug candidates, better understanding of the mechanistic processes of diseases and treatments, and more targeted personalized medicine becoming mainstream.
Author
Co-founder and CEO
Tiago Ramalho
Tiago holds a Master's degree in Theoretical/Mathematical Physics and a PhD in Biophysics from Ludwig-Maximilians University Munich. After graduation, he joined Google DeepMind as a research engineer. There he worked on a number cutting-edge research projects which led to publications in international machine learning conferences and scientific journals such as Nature. He then joined Cogent Labs, a multinational Tokyo based AI start-up, as a lead research scientist. In August 2020 co-founded Recursive Inc, and is currently CEO.