Accelerating Material Discovery using AI
AI2022-06-19
Material discovery is a research area with the potential to increase the sustainability of our economy in several ways. For example, by improving the efficiency of existing products and industrial processes, as well as enabling new applications and technologies that were not possible before.This is a crucial research field that often involves a lot of manual and time-intensive labor and trial and error by humans. Artificial intelligence could greatly help with this, by automating a lot of the work. We don't expect artificial intelligence to completely replace the end-to-end discovery pipeline, as we still need humans to guide its development and set the goals and understand the real world implications of certain developments. But we can see how it could help a lot with some aspects of the process.AI is beginning to gain a foothold in accelerating certain tedious parts of this system and automating experiments that often require trial and error.In the case of material discovery, there are two main areas where AI could be useful for the innovation pipeline: material property prediction and material synthesis.
Property prediction
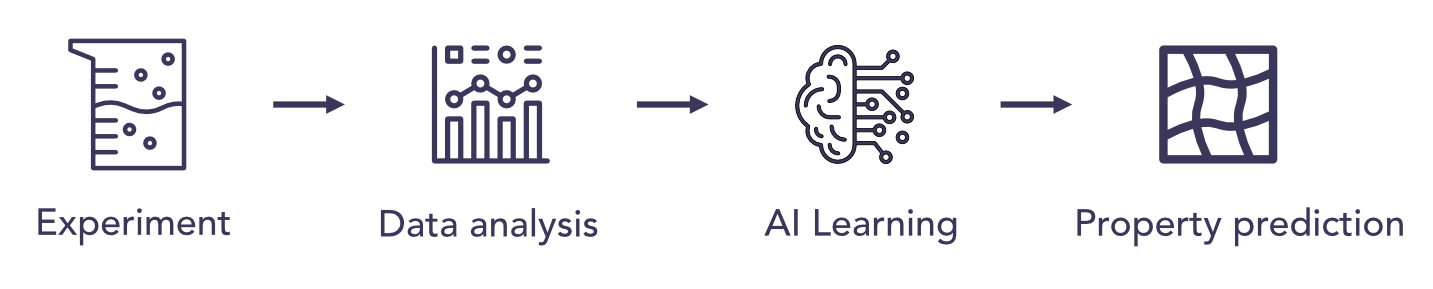
When material engineers have candidates for a novel material, they need to determine whether that candidate will be suitable for its intended purpose.
And to do so, a number of physical properties need to be characterized. For example, what is its pH? What is the temperature at which it reacts to other materials? What is its strength? What is the crystal structure of the material?
And of course, it is also important to predict what will be its actual effect when integrated in its final application. For example, in the case of a battery, what is its ultimate energy storage capacity? How fast can it charge and discharge? How many cycles can it withstand before degradation?
Predicting these properties often involves trial-and-error experiments, but by using data sets of other existing materials, we can train artificial neural network models that can generalize to other novel materials and can predict their properties both at the macroscopic level as well as at the microscopic level.
For example, at the macroscopic level, we could simulate the bulk structure of this material to determine its last transition temperature, tensile strength, melting points, density, viscosity, and energy capacity, and so on. At the molecular level, we can also predict its microscopic properties, such as lattice constant, band energy, or electron affinity, which are crucial for development of faster semiconductors or batteries and novel chemicals.
Material Synthesis
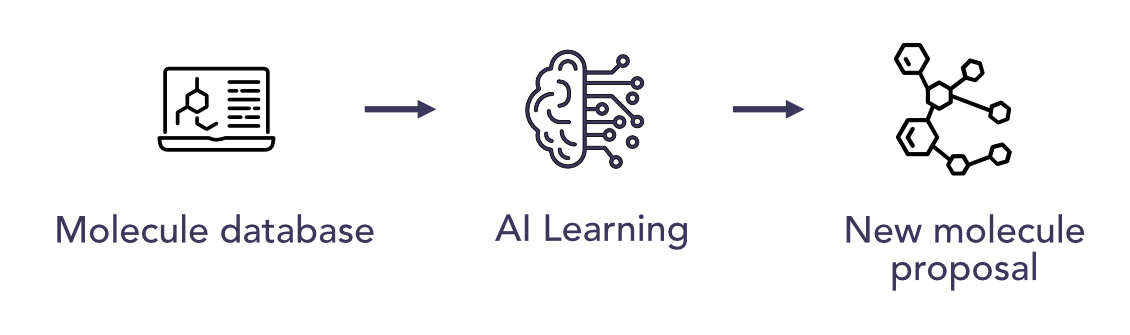
In addition, artificial intelligence can be used to discover and propose novel materials from scratch. For example, by filtering which chemical compositions are likely to form compounds, we can develop systems that recommend element combinations from a pool of elements or present ionic substitutions in existing materials for the discovery of new compounds.
We could also use these models to predict the crystal structure of a new material, so that we have a more guided exploration of which molecules are likely to have the crystal structure that we desire. An example from novel OLED systems shows that machine learning has been crucial to develop more optimized structures.AI can be also be used for process optimization. For example, to optimize the processing of material manufacturing, such as metal smelting. There are several key steps in this pipeline that could be accelerated by the use of AI, such as automating the analysis of the ore concentration, the processing of the ore, and the separation of the metals.
For example, by developing a model that can predict what mechanical properties an alloy will have given its processing parameters, we can optimize the processing parameters for the optimal mechanical properties in this alloy, and thereby improve the smelting method.
Additionally, AI methods have also gained a lot of popularity for the quantum mechanical simulation of these systems. When we're designing materials at the molecular level, quantum mechanical properties are very important and we need to have an accurate quantum mechanical description of the system. This is given by the density functional of the system, and often, these are very tedious and large calculations that have to be approximated numerically. And they may fail for very complex systems. However, by applying machine learning to the estimation of density functions, we can more quickly and more robustly predict the density functionals with higher accuracy.
Author
Co-founder and CEO
Tiago Ramalho
Tiago holds a Master's degree in Theoretical/Mathematical Physics and a PhD in Biophysics from Ludwig-Maximilians University Munich. After graduation, he joined Google DeepMind as a research engineer. There he worked on a number cutting-edge research projects which led to publications in international machine learning conferences and scientific journals such as Nature. He then joined Cogent Labs, a multinational Tokyo based AI start-up, as a lead research scientist. In August 2020 co-founded Recursive Inc, and is currently CEO.